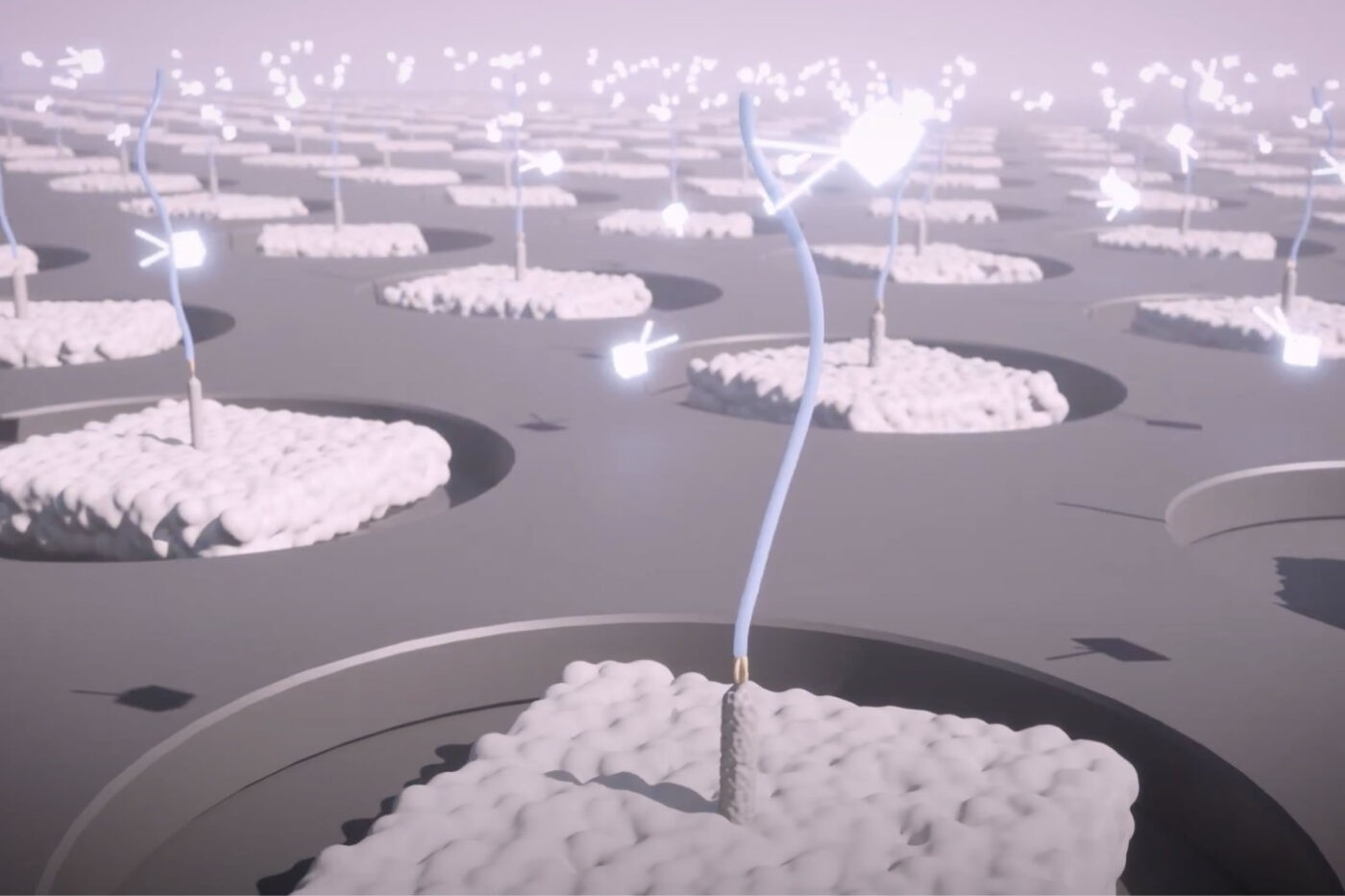
Next-generation proteomics technologies – Protein Identification by Short epitope Mapping (PrISM)
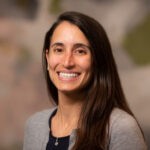
Jamie Rose Kuhar
December 13, 2022
Traditional methods of studying proteins cannot provide truly comprehensive data on the proteome, the full set of proteins in a sample with their associated abundances and locations. Even a relatively omics-scale method like mass spectrometry can only routinely measure a small fraction of the proteins in a cell.
Accessing largely untapped, comprehensive proteomic data for discovery proteomics and proteoform detection will enable scientists to better understand and manipulate cellular functions in health and disease. These proteomics advances will lead to better disease treatments and diagnostics in addition to high-yielding crops and novel means of protecting the environment.
Seeing this potential, many scientists have set out to create next-generation proteomics technologies. These technologies aim to deliver comprehensive data on the proteome from any sample of interest. Although many of these proteomics technologies are still in development, they should be available to researchers soon and will spur a proteomics revolution. In this series, we cover the concepts behind two kinds of next-generation proteomics technologies including:
- Next-generation protein sequencing
- Protein Identification by Short epitope Mapping (PrISM)
These posts are not intended to provide in-depth descriptions, but instead provide an overview of how these next-generation proteomics technologies work.
Today we cover Protein Identification by Short epitope Mapping (PrISM), the theoretical framework underpinning the Nautilus Proteome Analysis Platform. For more in-depth information on next-generation proteomics technologies see Alfaro et al 2021 and Timp and Timp 2020.
Next-generation proteomics through Protein Identification by Short epitope Mapping (PrISM)
With the founding of Nautilus, we set out to develop a proteomics technology that could deliver a simple but information-rich readout of the abundance of tens of thousands of proteins from just about any sample of interest with minimal sample prep and hands-on time. The theoretical framework underpinning our platform is called Protein Identification by Short epitope Mapping (PrISM).
With this technique, rather than use highly specific affinity reagents that bind to individual proteins, we make use of novel affinity reagents that bind to short sequences of amino acids with high affinity and low specificity. These probes stochastically bind to many different proteins. If one were to add just a few of them to a sample and monitor binding, they would not identify any individual proteins with confidence. Instead, with PrISM, we monitor how hundreds of these multi-affinity probes bind to individual proteins at the single-molecule level. This generates binding patterns for each protein molecule and then the patterns can be decoded by machine learning algorithms to identity of each protein molecule.
Alongside these multi-affinity probes, we have developed nano-fabricated chips with billions of landing pads for single-protein molecules. With standardized techniques, researchers can prepare their samples for automated loading onto the chips where individual proteins bind to each landing pad. We have also developed instrumentation that can monitor multi-affinity probe binding at each individual landing pad.
Thus, with our proteome analysis platform, we can flow hundreds of our multi-affinity probes over the chip sequentially and monitor binding patterns for each protein at the single-molecule level. The platform then uses machine learning algorithms to identify the proteins found at each landing pad following the PrISM framework. Because proteins are identified at the single-molecule level, we can quantify proteins in a sample by adding up identified proteins of the same species. The researcher analyze the results in various, insight-generating formats including a list a protein identities and their abundances.
Check out this video to learn more about how we quantify proteins on the Nautilus Platform
Pros and cons of PrISM as a next-generation proteomics technology
Pros
- Comprehensive proteome coverage – By isolating and repeatedly interrogating billions of single, intact protein molecules with a diverse array of multi-affinity probes that overcome the challenges of single-reagent sensitivity and specificity, PrISM can achieve a scale matching the proteome during broadscale protein analysis.
- Sensitivity – Single-molecule sensitivity enables PrISM to measure low abundance proteins and proteoforms with precision.
- Ease-of-use – A simple workflow from sample preparation to data analysis reduces time from sample to data. Researchers get insightful and information-rich results.
Cons
- Novel protein identification is challenging – Although the binding patterns identified by PrISM can be predicted from known protein sequences, PrISM cannot yet identify novel proteins. This may be possible in the future.
See our publications for more information on the framework for Protein Identification by Short Epitope Mapping (PrISM), and the development of our single-molecule protein arrays.
Next-generation proteomics technologies will power the proteomics revolution
The next-generation proteomics technologies described in this series will drive the proteomics revolution through applications in broadscale protein analysis, proteoform detection at the single-molecule level, and more. When these technologies are widely available, researchers will hopefully use them to make breakthroughs in basic research, healthcare, agriculture, and beyond!
N otes on next-generation proteomics comparisons
In the graphics portraying the technologies in this series, we provide qualitative assessments of proteome coverage and ease-of-use. While technologies with low proteome coverage are generally used for targeted experiments looking at a small number of proteins at once, medium coverage technologies can look at 100s to 1000s of proteins at once. Truly comprehensive, high proteome coverage technologies can analyze tens of thousands of proteins at once.
Low ease-of-use proteomics technologies generally require complicated, difficult, or customized sample preparation with a lot of hands-on experimenter time, and their data may be difficult to analyze or require bioinformatics support. High ease-of-use technologies employ simple, standardized sample preparation, include more automation, and provide simple, data-rich outputs. Medium ease-of-use technologies have some mix of these attributes.
In addition to assessing standard metrics like these, we also discuss some of the pros and cons of each next-generation proteomics technology. Many of the pros and cons discussed below are specific to the capabilities of the technology.
Importantly, the technology assessments here should not be viewed as definitive. Rather, they aim to help you think about how you can best leverage these technologies for your experimental goals.
MORE ARTICLES
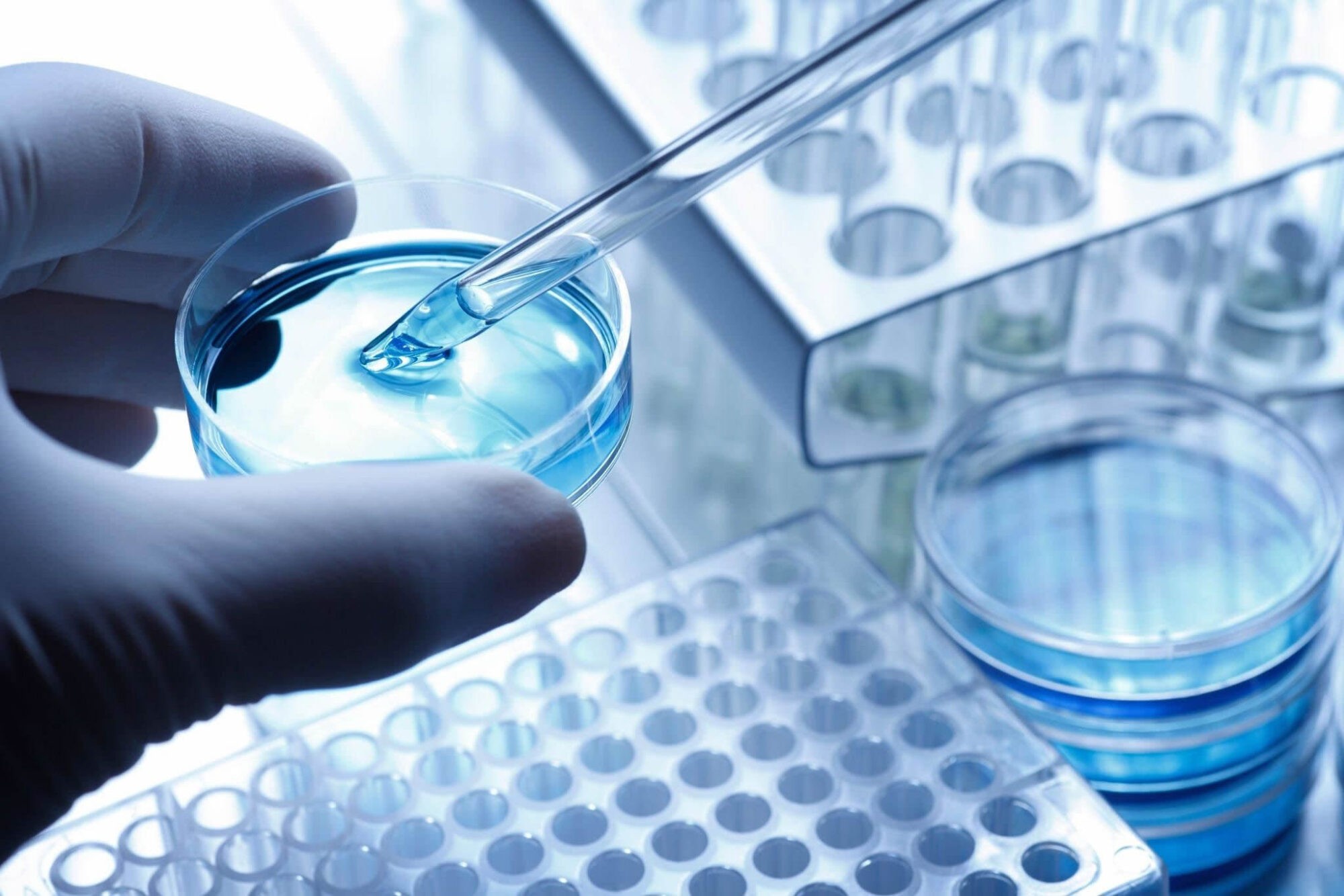