Multiomics is the comprehensive study of a biological entity through the combined use of various omics-scale methodologies. It enables researchers to holistically interrogate the molecular mechanisms of normal development, cellular responses, and disease. In this second post in our 3-part series on multiomics, we provide brief summaries of some of the methodologies used (and hopefully soon to be used) in multiomics research. If you’re planning on using multiomics techniques in your research, we hope this post can be a kicking-off point for your journey into this exciting field.
Be sure to check out our first post if you need a more general refresher on multiomics.
Proteomics
The human genome contains around 20,000 protein-coding genes, but our cells can produce far more than 20,000 distinct proteins. Proteins can be modified in multiple ways, including through alterations of mRNA transcripts and post-translational modifications of proteins, to create millions of variations known as proteoforms. The vastness of the proteome makes it a powerful, adaptable force in biology, even as its complexity makes it difficult to study. Proteomics technologies aim to measure all the proteins in a biological sample to get a comprehensive assessment of the proteome across cell types and disease states. As proteins carry out most cellular functions at the molecular level, some in the field have said that with truly comprehensive proteomics technologies, there is less need for full-blown multiomics studies.
Currently, scientists usually perform proteomic analysis studies using mass spectrometry. In a popular workflow, researchers start by fragmenting their proteins into peptides and separating the peptides using liquid chromatography (LC). As the peptides exit the LC column, they enter a mass spectrometer where they are given a charge, separated by their mass/charge ratios, fragmented, separated again, and the abundances of fragments with various mass/charge ratios are measured. These abundance measurements generate “spectra” that are like signatures for the original peptides and can be compared to databases of such signatures to identify their source peptides bioinformatically.
We and others are creating next-generation proteomic analysis tools that aim to provide a more comprehensive view of the proteome in a more accessible way. Following the PrISM framework, our platform is designed to repeatedly interrogate billions of intact protein molecules with a diverse set of novel multi-affinity reagents that bind to short sequences of amino acids shared by many proteins. Single proteins from a sample are first attached to their own landing pads on a 10 billion landing pad array. Then, the platform flows multi-affinity reagents over the array in many independent cycles, one reagent per cycle. High-resolution fluorescent imaging techniques determine which reagents bind to which proteins in each cycle and binding patterns of roughly 300 multi-affinity reagents are built for each protein. Machine learning algorithms decode the binding patterns to identify the proteins found at each landing pad. Because proteins are identified at the single-molecule level, protein quantification is performed by counting how many times a protein is identified.
See Alfaro et al 2021 for more information on “The emerging landscape of single-molecule protein sequencing technologies.”
Genomics
Genes provide the fundamental instructions for biology and understanding them can reveal incredibly important insights into a person’s health. Genomics is the study of the genome or the full completement of genes and other DNA sequences in an organism. For example, cancer genomics studies the genetic basis of tumor cell proliferation and evolution. It can help researchers understand cancer progression, develop biomarkers for different cancers, and design new cancer treatments. Genomics studies can be used in similar ways for a wide variety of diseases such as cardiovascular diseseas and neurological disorders like Alzheimer’s disease.
Genome sequencing today primarily makes use of “next-generation” DNA sequencing technologies (NGS). Using these technologies, researchers break genomes into many small fragments a few hundred base pairs in length, attach them to specially designed surfaces, amplify them, and synthesize complementary sequences using fluorescent nucleotides that can be imaged. This generates many short overlapping “reads” that must be computationally combined to generate full genomes. Recently, researchers have also been making use of technologies that read longer DNA sequences. Among other benefits, these “long-read sequencing” technologies help researchers sequence repetitive portions of the genome that are not amenable to short-read sequencing.
See the ThermoFisher and Illumina websites for more in-depth summaries of NGS.
Transcriptomics
Transcriptomics is the study of the transcriptome, or all the RNA molecules in a cell. This includes messenger RNA (mRNA) molecules that carry genetic instructions to ribosomes where they are translated into proteins, as well as other RNA that do not encode proteins like transfer RNA (tRNA), microRNA, small nuclear RNA (snRNA), small nucleolar RNA (snoRNA), and long noncoding RNA (lncRNA). Transcriptome analyses help researchers functionally annotate the genome, elucidate splicing, and measure differential expression of genes. In cancer research, transciptomics can identify cancer biomarkers as well as signatures of drug resistance, the tumor microenvironment, and neoantigens. Single cell RNA sequencing (scRNA seq) in particular can help researchers discern intratumor heterogeneity with precision. This can help reveal the molecular mechanisms of cancer progression at high resolution.
There have been many technologies developed to study and quantify transcriptomes. Currently, researchers largely leverage DNA sequencing technologies to identify RNAs expressed in an organism. In a generalized workflow, they isolate RNA molecules from their cells or tissues of interest and reverse transcribe the RNAs into so-called “cDNAs” using reverse transcriptase enzymes. These sequences are fragmented into smaller (a few hundred base pair) pieces that are amplified and sequenced with next-generation sequencing platforms. Bioinformatics tools are used to map these short sequence reads back to the genes that encode them.
Researchers can also use microarrays to identify the RNAs expressed in a sample. Here, cDNAs are hybridized to oligonucleotide probes of known identity on an array. Researchers can use signals resulting from hybridization to both identify and quantify the expressed RNAs.
With either technology, comparative analyses of gene expression across samples can associate gene expression with cellular functions or states. This is useful information, but RNA expression rarely correlates well with protein levels and doesn’t necessarily identify pathways active in cells now.
See Lowe et al 2017 for a review on “Transcriptomics technologies.”
Epigenomics
Epigenetics is the study of heritable changes to gene expression that don’t stem directly from mutations to DNA. Epigenomics aims to study all the DNA and protein modifications that impart this heritable regulation of gene activity. These changes usually come in the form of molecular tags added to DNA (e.g. methylation) or to proteins associated with DNA (e.g. acetylation of histones).
Researchers can use various techniques to identify epigenetic modifications. For example, in bisulfite sequencing, chemical treatment converts un-methylated cytosines in DNA to uracil. Next-generation sequencing techniques are then used to sequence the DNA. Any cytosines that remain in the final sequence must be methylated. DNA methylation can also be studied using affinity-enrichment. In this technique, the methyl-binding domain the of MeCP2-protein can be used to selectively isolate methylated DNA from samples. Later this DNA can be identified by sequencing.
ChIP-seq is another common technique of epigenomics. It is used to determine which proteins are associated with particular DNA sequences. In this technique, DNA binding proteins are isolated with specific antibodies against them. This is followed by dissociation of the proteins and DNA sequencing. This reveals, in a genome-wide way, what proteins are bound to which DNA sequences. One common application of ChIP is to analyze the binding of transcription factors and proteins important for maintaining chromatin.
See Li 2021 for a review on “Modern epigenetic methods in biological research.”
Metabolomics
Metabolomics is the study of the metabolome, or the collection of all the small molecules (metabolites) present in an organism. Metabolites form a broad category that includes lipids, sugars, organic acids, amino acids, and more. Abundant metabolites in biological specimens may be directly related to the function of that specimen or to pathogenic mechanisms. Studying the metabolome provides a broad overview of the molecules produced by a cell or organism. This, in turn helps researchers understand such things as biological responses to infection or other biological perturbations. Metabolomics encompasses a broad range of molecules and can provide a good snapshot of the active processes in a cell or organism.
For metabolomics studies, a sample is often dissolved in a solvent that is good at solubilizing a particular type of metabolite. The dissolved sample is then fractionated using a variety of techniques such as gas chromatography or liquid chromatography. Sample fractions containing metabolites are then analyzed (often inline) on a mass spectrometer. The time it takes for a given metabolite to enter the spectrometer as well as the mass/charge ratios detected in the mass spectrometer are used to identify and quantify the metabolites in the sample.
See David and Rostkowski 2020 for a review on “Analytical techniques in metabolomics.”
Discerning insights from multiomics data
Combining the data generated from all these techniques is no easy task. It requires bioinformatic analysis and expertise. Increasingly, researchers are also using AI to discern patterns in their data and generate inferences about relationships between omics and phenotypes. In the next post in this series, we’ll provide examples of the insights that can be gleaned from these analyses.
Check out this episode of the Translating Proteomics podcast to learn how we can leverage multiomics to study “Biology in Space and Time”
MORE ARTICLES
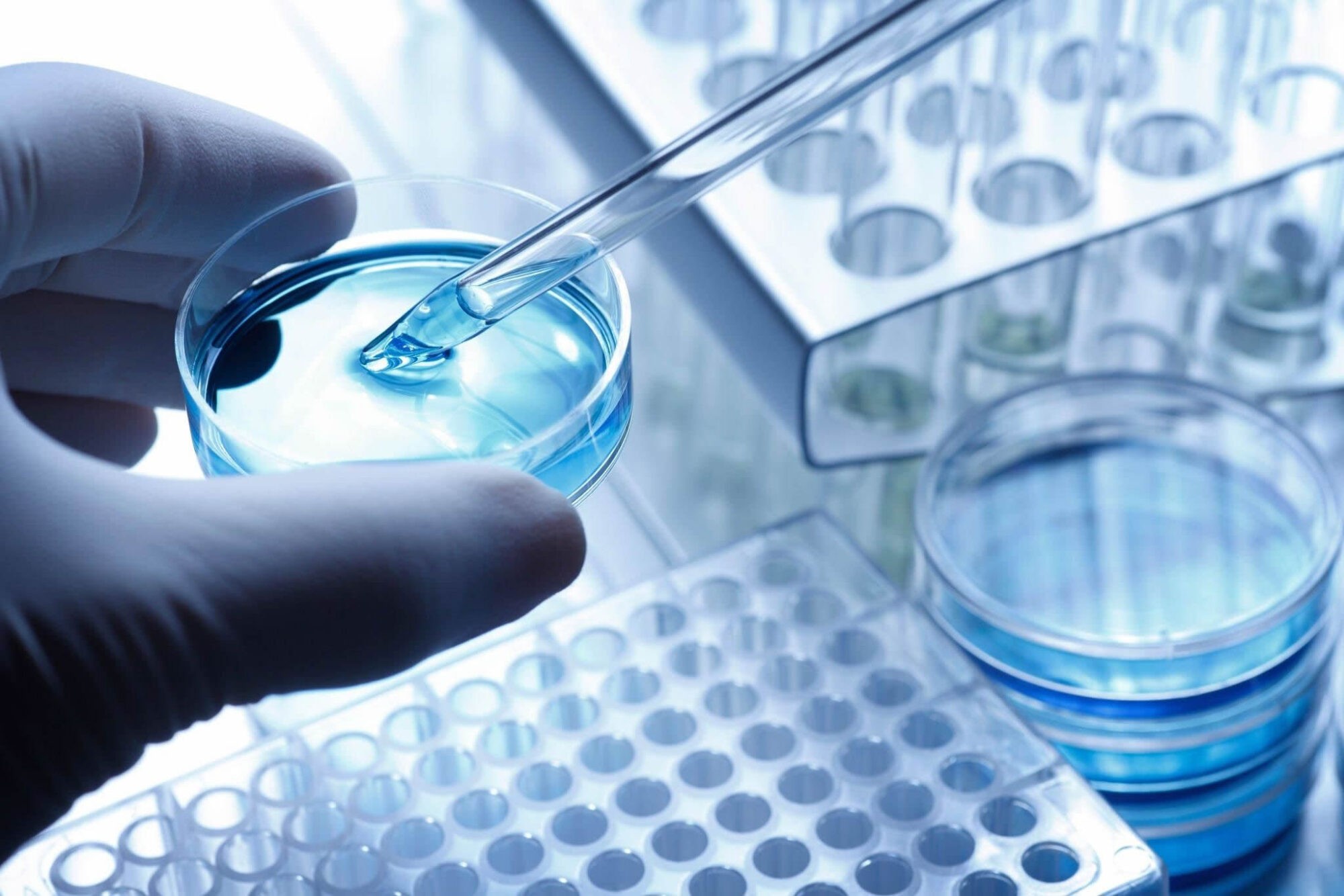